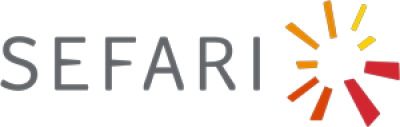
Work Package Agricultural systems and land management
Introduction
In the digital era the potential use of integrated “big data” has the potential to revolutionise agriculture in the way it has done for many other industries. Farmers and supply chain stakeholders need to understand and value technology, in order to make decisions more quickly and methodically and not lag behind the innovative approaches. The potential application of data driven science in farming is not only about primary production, but also have a significant role in enhancing the efficiency and sustainability of effectiveness of the entire supply chain. The opportunities of data driven solutions explored within this RD include integrating sensor data across farming platforms; within and across farm production prediction models and integrating primary production data with national data sets including supply chain and weather databases.
Aim of Research
This RD will involve the development of tools and strategies to promote the increased use of data from across agricultural supply chains and industry networks, for management and feedback, in order to improve efficiency across the agri-food industry. We will focus on developing methodologies to help quantify and communicate the uncertainties resulting from pooling data across the supply chain. We will develop crop and livestock case studies building links with RDs 2.3.9 & 2.4.1 (barley) and WP2.2 & 2.4 (beef supply chains). We will work with cattle industry data to identify data that could be shared across the supply chain (public and private sources) and the potential benefits in doing so. We will also work with the barley industry (which is also relevant to the beef industry), by linking with the Home Grown Cereals Association (HGCA) on focusing improvement of business opportunities by providing tools to measure and interpret the impacts of climate change on barley production and quality for both animal and industrial purposes.
Progress
Results have demonstrated that altering the efficiency of beef animals when they are growing and finishing within the farm gate can have supply chain benefits in terms of reducing the costs of production and the environmental footprint of beef production. This can help to inform supply chain managers as to key targets for farmer incentivisation to improve across-supply chain performance metrics. Work focussed on integrating individual beef animals' records of feed intake (~2000 animals) and subsequent final carcass weights, age at finishing and grading, including price paid. Analysing these data highlighted that the value of faster growing animals compared to average of the population for days to slaughter based on feed energy saved through earlier slaughter was estimated to be worth £0.20 worth of feed saved per day during the growing and finishing phase.
We have further explored how animal performance data can be merged with animal mounted devices to help predict attributes of animal performance from the 'wearables' alone. Work in the reporting year has extended statistical methodology and modelling so that it can be applied to data obtained from deploying multiple sensors simultaneously. This has specifically been applied to the methods used for live weight data and automatic milk yield data to evaluate the performance of the methods against auxiliary data.
Linking to RD 2.3.3 we have coded and refined disease modelling to integrate using national livestock movement data to inform development of national scale dynamic models of livestock disease.
Building on data collected and models developed earlier in the programme in the reporting year we have produced maps of barley production data. These spatial and temporal patterns have been compared to observed barely data for the whole growing area of Scotland across soil types and weather conditions over 22 year period (27,500 combinations of soil/weather). The model has been set up and the spatial map, overlaid using the Scottish Soil Database and the Barley growing area
Highlights:
- Evidence from this and linked RDs on the potential role of efficiency improvements in meeting our carbon targets from agriculture presented to the Environment, Climate Change and Land Reform Committee (Nov, 2018).
- These results have been communicated with farmers at the British Cattle Breeders Club (Jan 2019) to demonstrate the economic value of improving feed efficiency in growing beef cattle, which can be used to inform management decisions.
Building on results from year further discussions took place with red meat industry stakeholders (e.g., processors, auctioneers/marts and supply chain stakeholders) to explore how data can be mined to identify key supply chain (in)efficiencies. Much of this focussed on displaying impact of different ages at slaughter on overall efficiency. Linking with the Beef Efficiency Scheme herds, the age at slaughter varied from 1-3 years with a mean of 1.75 years across all breeds. The most important influence on age of slaughter was shown to be finishing herd, which is a proxy for finishing system, followed by birth herd and sire breed. This highlights that at a phenotypic level that finishing system type is a key driver of the efficiency at which the beef animals reach slaughter. Extrapolating to economic performance and GHG footprint of red meat production, results showed that a finishing age of 26 months or older was a cost to the production system rather than generating a profit. The initial results have shown that reducing age at slaughter (30 to 18 months) can reduce GHG emissions by up to 15%.
Building on data sets identified in year one we focused on the use of automated sensor data as an "early warning" system for detecting adverse health and/or welfare events in farm animals. A prototype methodological framework for using statistical models (linear mixed models) to describe patterns of variation in sensor data, and hence to detect departures from the background level of variation that would be expected in the absence of adverse events, was developed. Methods to validate “early warning” systems was also developed. The prototype early warning methodology was applied to a particular form of sensor data collected from a dairy research herd (IceTags, which record movement using an accelerometer), and has been used to try to identify both adverse health events and the occurrence of fertility (heat) events.
A data driven model of the whole barley growing area of Scotland was run simulating yield under historical climate weather at a spatial scale. A total of 2,500,000 simulations were run looking at difference soil-weather combinations over different timescales and weather scenarios. This work has been done to provide a baseline for simulating production of barley under projected climate and for yield forecasting tool. The nature of the work and its output has attracted the attention of the Whisky Industry. The next steps will be to finalise the work and run it with climate projections to quantify the changes in future barley production and quality.
Highlights:
- Material prepared and presented to various (inter-)national industry related meetings including the use of national data sources to model female fertility in beef cattle at the 2017 International Committee for Animal Recording
- Farmer/industry information generated and presented at the launch of national carcass trait evaluations in collaboration with AHDB (May 2017).
- Results on the merging of carcass data with Beef Efficiency Scheme data shared in report with farmer participants.
- Work on impacts of weather on barley production in Scotland presented at the Climate Impacts Conference in Potsdam (Germany).
As the focus of this RD is data driven solutions for agriculture much of the first year focussed on securing, collecting and collating data and methodologies for the integration and analysis livestock and cropping industry data from a range of sources.
In beef production the objective is to develop of tools and strategies to promote the increased use of data from across agricultural supply chains and industry networks, for management and feedback, in order to improve efficiency across the industry. Modelling industry data will enable the generation of information and tools to improve efficiencies of supply of beef to abattoirs, and identify novel approaches to monitor the supply and wastage profiles. Based on analyses of government databases (British Cattle Movement Survey) and industry carcass data we were able to classify carcasses into different "fate" categories (i.e., if the carcass had elements condemned due to diseases such as liver fluke). In analysing these data we identified that carcasses with liver fluke condemnation took approximately 30 days longer to reach a set carcass weight and thus is a cause of inefficiency in the supply chain.
Sensor data can be used to develop models and prediction of attributes of animals, farms and the environment. However the data can be complex and therefore optimal data processing and statistical methodologies need to be identified. Key gaps relating to existing methodologies for the analysis of sensor data were identified, and methods to fill these gaps explored. Relevant datasets to test these methods have been identified in consultation with a range of scientists working with sensor data.
Predicting yield implication of extreme events in agriculture is critical. Linking with activity in 2.3.9 we will use a soil-crop-atmosphere model to study the impacts of weather and climate on barley production and quality. Extensive data on crop performance, soil and weather data on agricultural land in Scotland were collected and collated as model inputs. Associated databases and data queries were developed, to support future modelling of how weather impacts on performance of Scottish cropping systems.
Highlights:
- Key messages and presentations were developed and presented at a range of (inter-) national events including at Livestock Gentec Conference (Canada, October 2016) and Sheep Breeders Round Table on analysing national carcass traits and economics of age at slaughter.
Future Activities
Going forward we will use amassed data and models to mine for key information for animal/crop managers and supply chain stakeholders to improve the efficiency and sustainability of their production systems. For beef this will focus on analysis of the BES data in relation to the incidence and impact of key diseases as recorded by BES participants to examine the post farm gate impact of disease on efficiency of beef production in Scotland. Work will continues on the development of robust statistical analysis of data generated from sensors to help provide useful and real time management messages for farmers. A range of climate change events (e.g. drought spells, excessive rainfall) will be modelled to map out impacts on barley production and quality. This will produce spatial maps of Scotland, Figures showing cause-relationships of the impacts of climate extremes due to climate change in Scotland.
Selected Outputs
Analysing carcass trait performance across a lifetime.
Using Video Image Analysis to help improve efficiency in primal beef production via genetics
Johnes disease linked to lower production in dairy cattle
Crop model improvement reduces the uncertainty of the response to temperature
Designing future barley ideotypes using a crop model ensemble
Data-driven risk assessment from small scale epidemics
Impact of herd management on efficiency in beef production
Hot spots of wheat yield decline with rising temperatures
Estimation of under-reporting in epidemics using approximations
Weather impacts on barley yield and malting quality in Scotland